About Us
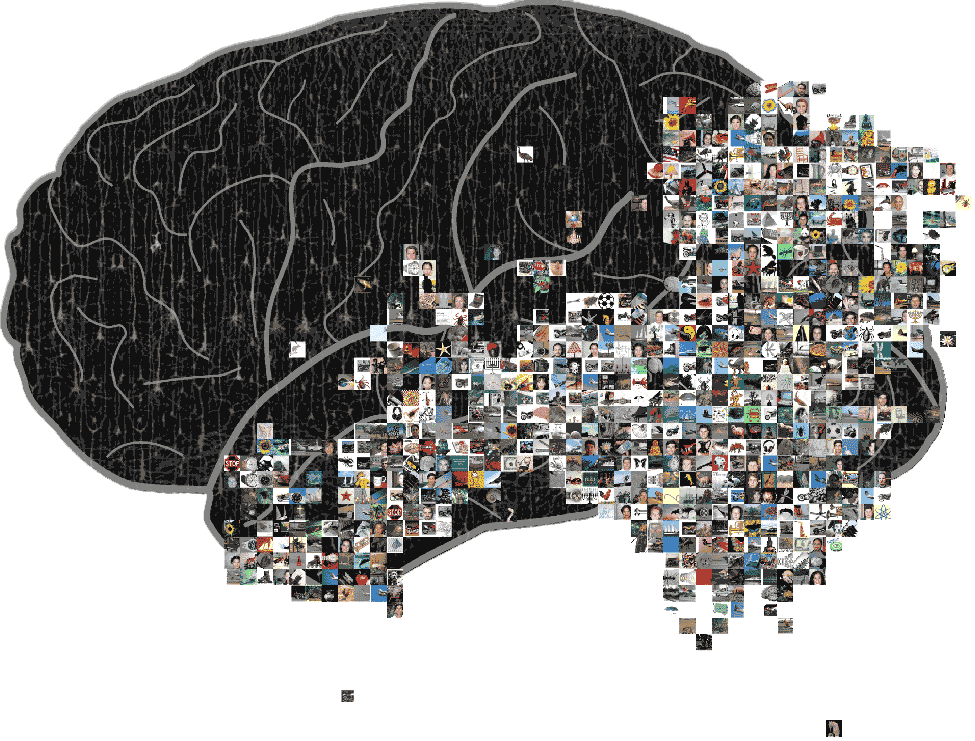
Our Lab
Our lab investigates how cortical circuits interact to form transformation-invariant object representations that can guide behavior. Natural environment contains large number of objects with overlapping sensory input, and our brain is capable of using information from different sensory modalities to extract their identities with ease. Yet, despite extensive research in the last few decades, we are still far from having a complete understanding of how the brain creates untangled object representations. If we understood how cortex achieves this extraordinary ability at the algorithmic level, this would represent a significant advance in our understanding of brain computation in general. To address this question, we combine advanced imaging techniques for recording neural activity with high-throughput behavioral training and computational modeling to study how the activity of large neuronal populations across different cortical regions enables behaving animals to identify and isolate objects in different contexts.
What are we interested in?
The brain tries to build an internal model of the environment based on information from multiple sensory systems, in order to guide future actions. Every day, we see thousands of unique objects with many different shapes, colors, sizes and textures. Each one of these objects can generate a virtually infinite number of different images on our retinas, depending on the conditions we view it under. In spite of this extraordinary variability, our brain can recognize objects in a fraction of a second and without any apparent effort. To accomplish this computationally challenging task, a large part of our brain is dedicated to processing all the available information from the environment and extracting and isolating object identities. Fundamentally, we want to understand how different circuits in cortical areas coordinate to perform this complicated computation.
What methods do we use in our research?
We use a combination of computational, behavioral, electrophysiological, two-photon and widefield imaging, and optogenetic techniques. We train mice to perform object discrimination tasks that require multiple kinds of sensory information. Importantly, we study the neural responses of awake animals to sensory stimuli both during passive tasks, and during behavior. Finally, we use computational models, to understand how the activities of large number of neurons generate invariant object representations, and to try to infer how different circuit architectures might underly this ability. The models give us greater insight into potential mechanistic interpretations and guide our next experiments.
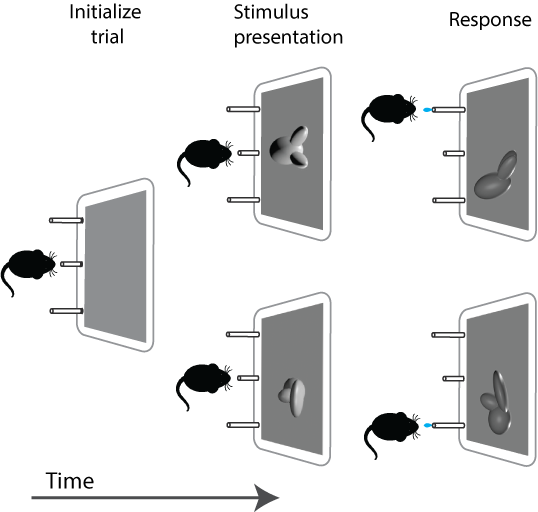
What are some of the questions we are studying?
Mouse object recognition: Even though mice are considered nocturnal animals, there have been plenty of studies showing that mice can perform complicated visual tasks. However, due to the difficulties in training these animals on complicated tasks, there has been limited research in this area. A first step is to try to answer the question: “What are the visual object recognition capabilities of mice?” Can we develop tasks and objects that are easy for these animals to learn? How do the animals use this information to navigate? With the help of our high-throughput behavioral home-cage training system that runs on low-cost Raspberry Pi’s we can overcome the limitations of training individual animals and explore the parameter space for difficult tasks. Additionally, this automatic training method provides a good screening method for various cognitive deficits.
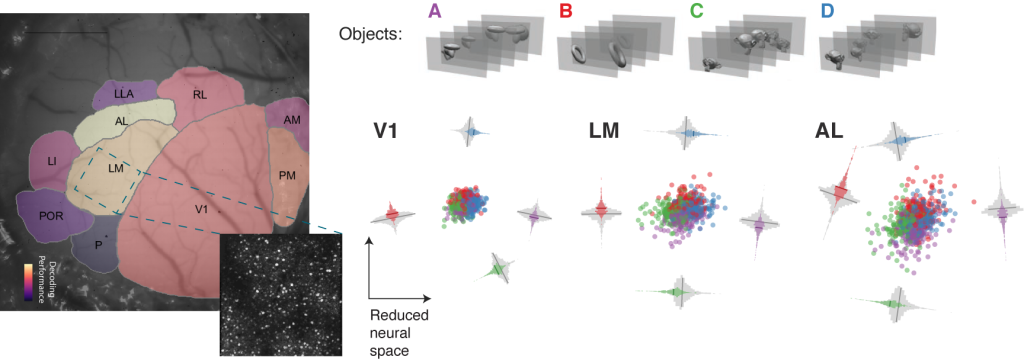
Object representations across the visual hierarchy: : One of the primary areas of research in our lab is to identify the visual areas that are critical for object recognition. How do the neural representations evolve across the visual hierarchy and how do cortical circuits across these areas interact? Does visual experience alter these representations? How are representations of multiple objects multiplexed across populations of neurons?
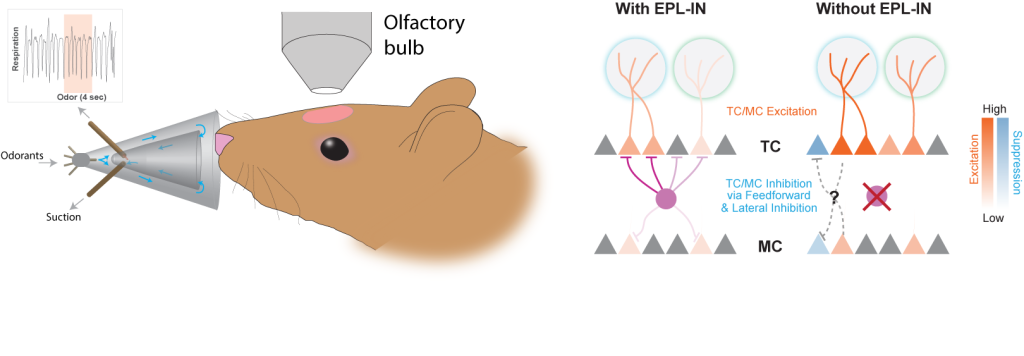
Multimodal object recognition: Objects can elicit a plethora of sensory
stimulation that may involve one or more senses; for example, a cat in the
world has visual, olfactory, auditory, and somatosensory features. Our brains
have evolved to be able to assign these diverse features correctly to a single
object base on constancy and correlation in the sensory input. Despite the fact
that this is a central characteristic of our ability to identify objects, most
studies on object recognition do not use such a rich stimulus set of
information or include the dynamics of real existing objects. We are using
multisensory information (olfactory, visual, auditory) to define objects that
animals have to discriminate, and we are investigating the cortical areas and
neural activity that are necessary to integrate these sensations into a single
representation of an object
Contact us
E-Mail: frouman@imbb.forth.gr
agapi_ntretaki@imbb.forth.gr
Telephone: +30 2810391057
Address: ITE-FORTH, N.Plastira 100, 70013
Heraklion, Crete, Greece